Research
- Home >
- Technologies >
-
Using informal data for credit scoring users without traditional bank credit his ...
RESEARCH
Using informal data for credit scoring users without traditional bank credit history
study addresses this challenge by developing tailored credit scoring models for informal merchants using supervised learning techniques. Leveraging data from a financial technology company in Lesotho (Southern Africa), we applied logistic regression and support vector machines to predict the likelihood of
loan defaults among merchants. Our methodology involved the evaluation of six logistic regression models and twelve support vector machine models, assessing their effectiveness in default prediction. The results provides a robust tool for more accurate assessment of creditworthiness, reducing the
risk of lending to potential defaulters. The study underscores the potential of supervised learning methods to create impactful financial solutions and suggests a pathway towards narrowing the financial inclusion gap in the informal African economy. This approach not only aids in risk reduction for
lenders but also empowers a critical segment of the economy by enabling better financial support and growth opportunities for informal sector merchants.
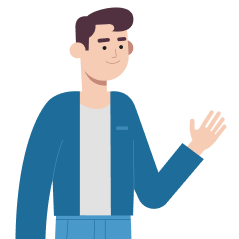
To view more project details and submit an expression of interest
Explore more projects
Solutions to Business Technological Challenges
-
-
-
RESEARCH
08 Oct 2023
Dr. Guy TanentzapfVancouver
- stem cells
- blood stem cells
- immune cells
- stem cell activation